Building an AI generated game with Stable Diffusion and data from Wikipedia
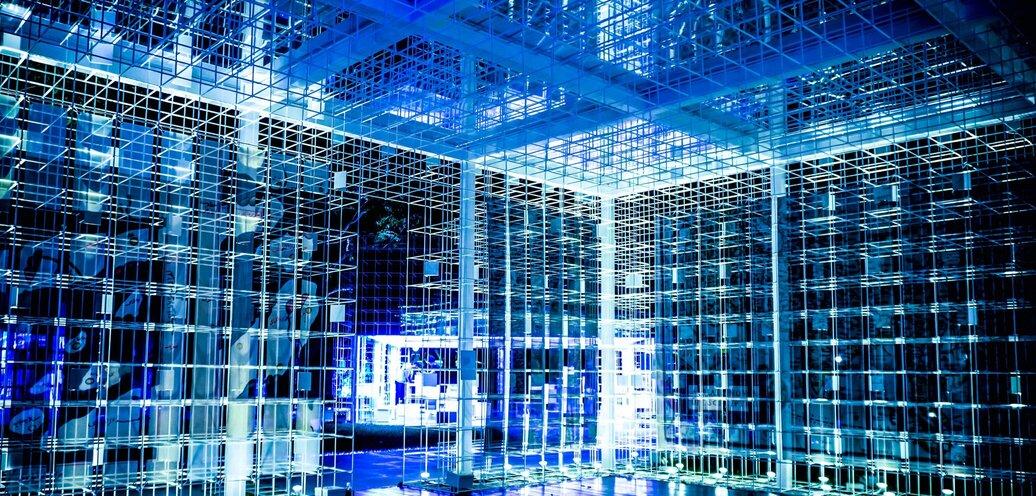
Last week I released a game called Doodle:ai.
In the game you're shown AI generated images and you have to guess the Wikipedia topic it used to create the game.
Doodle:ai has had thousands of players since launch and I thought I would share how I built it.
The idea
There has been incredible progress in generative AI models over the last 3 months and I wanted to build something with it.
I had this idea to automatically create Google Doodles based on Wikipedia topics.
As I was building this Google Doodle generator tool, I thought it might be fun to see if I could randomise the output a little and guess what the AI had chosen for a day.
It worked as a game so I took that direction.
The Architecture
The game runs off pre-generated puzzle data.
The backend is a NestJS server which exposes endpoints to queue up puzzle generation jobs and store the output in a Digital Ocean Spaces blob store.
The frontend is a React app which uses the Digital Ocean Spaces store to get the daily puzzle.
The high level process for generating a puzzle
- An administrator (Me) queues up a puzzle generation job for a date range. These jobs get put in a Bull queue by a NestJS controller.
- The Bull queue workers retrieve and process daily topics from Wikipedia.
- The topics are filtered and cleaned up to remove topics that are not suitable for a game.
- A random topic is chosen and the worker requests the summaries for Wikipedia links and articles relevant to the topic.
- The summaries are combined into an essay and sent to Azure NLP for analysis.
- The NLP analysis is filtered and mapped to useful combinations for prompt generation.
- Lots of prompts are generated and 4 random prompts are chosen.
- The prompts are sent to stable diffusion to generate images.
- The images and puzzle data are packaged and sent to Digital Ocean Spaces.
- A customer opens the game and the puzzle data is retrieved from Digital Ocean Spaces.
Topic selection
The first step is to select a topic for the game. Wikipedia is a relatively high quality source of well organised trivia.
I use the Wikipedia API to get a list of topics for a given day. I then filter the topics to remove topics that are not suitable for a game.
I originally accessed the wikimedia api directly. The first few games used this data.
But Wikitext is extremely difficult to parse so I have changed to use the wtfWikipedia node library. This returns a structured javascript object and is much easier to work with.
See an example of wikitext below. It's so hard to parse, thankfully we have open source parsers like wtfWikipedia.
{{Short description|Capital city of Ontario, Canada}}
{{About|the city in Ontario}}
{{Redirect|City of Toronto|the city's government|Municipal government of Toronto}}
{{Pp-move-indef}}
{{Pp-vandalism|small=yes}}
{{Use Canadian English|date=July 2014}}
{{Use mdy dates|date=April 2021}}
{{Infobox settlement
| name = Toronto
| official_name = City of Toronto
| settlement_type = [[List of cities in Ontario|City]] ([[List of municipalities in Ontario#Single-tier municipalities|single-tier]]){/* Consensus see: [[Wikipedia talk:WikiProject Ontario/Archive 1#City infoboxes: "tier" or "conventional" municipal statuses (or both)?]] */}
Once the Wikipedia pages are parsed, I filter out topics that are not suitable for a game. This is generally topics that I feel will result in images that are too visceral or offensive like mass shootings or terrorist attacks.
The final step is simply choosing a random topic as the correct answer for the game.
Entity Categorisation
The game requires keywords for guesses and keywords for image generation prompts. To create these keywords I build an "essay" from the topic content and any adjacent Wikipedia topics.
public async getSummariesOfPages(pageNames: string[]): Promise<string[]> {
const summaries = (await wtf.fetch(pageNames, {
lang: "en",
// eslint-disable-next-line @typescript-eslint/naming-convention
follow_redirects: true,
})) as WtfWikiDocument[];
const parsedWikiPages = summaries && summaries.map((x) => x.json());
this.logger.log(
"summaries",
util.inspect(parsedWikiPages[0], false, 8, true)
);
// continue to parse and filter the data
// ...
// ...
}
This essay is sent to azure NLP which returns a list of detected entities. This entity list is passed to the next stage.
const client = new TextAnalyticsClient(
this.config.url,
new AzureKeyCredential(this.config.apiKey)
);
const results = await client.recognizeEntities([text]);
this.logger.log("Text analysis results", results);
// map the results to a list of entities
// ...
Prompt Creation
The difficult part of using AI generation tools is creating prompts.
I have gone through four major refactors of my prompt generation process. I have to provide spoilers for these games to explain the prompts. Sorry about that!
Play all the games first before reading this if you prefer.
1. Simply add all categorised entity keywords to 4 fixed prompts
This method resulted in some very interesting images but maybe 1/3 were usable for the game and they were all quite similar.
Example: "watercolor, 1995 gulf of aqaba earthquake, mercalli intensity scale, gulf, of aqaba earthquake, sinai peninsula, saudi arabia, tsunami"
Play this game here: https://doodleai.darraghoriordan.com/?gamedate=2022-11-22T12:00:00.000Z
2. Split entities randomly across a few random prompts
I tried randomising the keywords and selecting the first half for one prompt and the second half for another prompt and so on. This was actually OK but there were still issues where prompts that were suitable for portraits were used for landscapes and vice versa.
Example: "denny party, seattle,by Josef Thomas, matte painting, trending on artstation HQ"
Play this game here: https://doodleai.darraghoriordan.com/?gamedate=2022-11-13T12:00:00.000Z
3. Select the entities with best confidence scores and randomly join them
I tried only selecting the top 4 entity confidence scores. These were OK but categorisation confidence is not the same as topic relevance, so this often missed good, relevant keywords.
Example: "Donald Johanson, Australopithecus afarensis, Lucy (Australopithecus), The Beatles, depth of field, photograph, Polaroid 600 Instant Film"
Play this game here: https://doodleai.darraghoriordan.com/?gamedate=2022-11-24T12:00:00.000Z
4. Split entities in set patterns, use specific lists of prompts suitable for the category
This is the current method I use. It results in mostly relevant images and a good variety of images.
Example: "a hyper realistic professional photographic view picture of Richard Seddon, New Zealand photographic filter unreal engine 5 realistic hyperdetailed 8k ultradetail cinematic concept art volumetric lighting, fantasy artwork, very beautiful scenery, very realistic painting effect, hd, hdr, cinematic 4k wallpaper, 8k, ultra detailed, high resolution, artstation trending on artstation in the style of Albert Dros glowing rich colors powerful imagery nasa footage drone footage drone photography"
Play this game here: https://doodleai.darraghoriordan.com/?gamedate=2022-11-28T12:00:00.000Z
With this prompt generation method I split the entities into some custom groupings and then select a random prompt from a list of prompts suitable for my pseudo-categories. e.g. I make sure Persons are used for portraits and Locations are used for landscapes.
Azure NLP categorisation is pretty good. There is a confidence and often a sub category included for a single entity.
These are the main categories doodle:ai uses.
Person - Names of people.
PersonType - Job types or roles held by a person
Skill - Skills or abilities
Event -Natural - Naturally occurring events.
Event -Sports - Sporting events.
Location - GPE - Geographic and natural features such as rivers, oceans
Location - Structural - Manmade structures. e.g. ships
Organization - Medical
Organization - Stock exchange
Organization - Sports
I link these together to make prompts so things like
Person + Location - GPE Event - Sports + Organisation - Sports
const removeUnwantedCategories = nlpEntities.filter(
(x) => !unwantedCategories.has(x.category)
);
const geoLocationEntities = removeUnwantedCategories.filter(
(x) => x.category === "Location" && x.subCategory === "GPE"
);
const manMadeEntities = removeUnwantedCategories.filter(
(x) => x.category === "Location" && x.subCategory === "Structural"
);
// more of these
// ...
// then map them to entity combinations and random prompts
const manMadeAtLocations = this.createPrimaryWithRandomSecondaryPrompts(
manMadeEntities,
geoLocationEntities,
locationPromptTemplates
);
//
const generatedPrompts: string[] = [
...manMadeAtLocations,
// and many more of these
// ...
]
.sort(() => Math.random() - 0.5)
.slice(0, requiredNumberOfPrompts); // then grab just 4 and return
return generatedPrompts;
Linking entities seems to result in better output so I will likely stick with this method and focus on improving the actual prompts.
Unfortunately, there is no way for me to link things like "person" and "location" accurately in the context of the topic so sometimes there are very weird results due to my random process. e.g. I might end up with "Ghandi, England" where instead "Ghandi, India" would result in a more coherent image.
Another issue is that sometimes the adjacent articles are too far removed from the main topic to be useful but I have no way to tell relevancy or adjacency as a score at the moment. So I randomise the prompts and hope for the best.
I've recently added in one image that is only the keywords to see what the AI does with it. It is usually pretty bad but sometimes it is quite interesting.
I'll keep tweaking this generation process. It's fun to see what works and what doesn't.
There is an incredible amount of randomness in this process.
- The topic is chosen at random for a given date
- Entities for my pseudo-categories are chosen at random
- The order of the combined entities in prompts are random
- I create 30-40 prompts per game and choose only 4 of them
- Stable diffusion is passed a random seed for each prompt
I have no idea what the output will be until I see it, and I can never recreate a game.
Stable Diffusion
I'm not doing anything very special with Stable Diffusion for Doodle:ai. There are heaps of great articles on how to setup and run stable diffusion on a PC.
One thing I did change from the default is removing the nsfw filter because it's too eager.
A difficult thing was running the conda environment required for python from a web server.
First I had to wrap the nodeJS spawn call in a Promise.
const execPromise = util.promisify(exec);
// ...
public async execAsPromised(
command: string,
commandArguments: string[],
cwd: string,
shell?: string
): Promise<string> {
this.logger.warn(`Executing cli command '${command}' in '${cwd}'`);
this.logger.warn(
"Note: Do NOT allow user input as parameters for 'execAsPromised' as inputs are not sanitised and a hacker could take over your system."
);
const result = await execPromise(
`${command} ${commandArguments.join(" ")}`,
{
shell: shell || "/bin/bash",
cwd,
}
);
return result.stdout;
}
Then I call the txt2img
script combined with conda
rather than launching conda as an environment first.
import { spawnPromise } from "./SpawnPromise";
import { CoreLoggerService } from "@darraghor/nest-backend-libs";
import { Injectable } from "@nestjs/common";
import os from "os";
import path from "path";
import { execSDPromise } from "./ExecPromise";
@Injectable()
class StableDiffusionRunner {
constructor(private readonly logger: CoreLoggerService) {}
// eslint-disable-next-line @typescript-eslint/require-await
async run(prompt: string, puzzleDate: Date) {
this.logger.log("running sdiff", prompt);
// create random integer seed as string
const seed = Math.floor(Math.random() * 1_000_000_000).toString();
return execSDPromise(
"conda",
[
"run",
"--no-capture-output",
"-n",
"ldo",
"python",
"-u",
"optimizedSD/optimized_txt2img.py",
"--prompt",
`"${prompt}"`,
"--n_iter",
"1",
"--n_samples",
"1",
"--H",
"512",
"--W",
"512",
"--turbo",
"--seed",
seed,
"--ddim_steps",
"50",
"--skip_grid",
"--outdir",
`./output/${puzzleDate.getUTCFullYear()}/${
puzzleDate.getUTCMonth() + 1
}/${puzzleDate.getUTCDate()}`,
],
path.join(
os.homedir(),
"projects",
"personal-projects",
"stable-diffusion"
),
"/bin/bash"
);
}
}
export default StableDiffusionRunner;
I do occasionally run out of memory when generating images if I'm doing other work on the PC - not VRAM, just regular RAM.
I "only" have 16GB. I guess I should have gone for 32GB! If I run generation while I'm not using the PC it works for hours no problem.
The game engine / frontend stack
The frontend is based on a stack I use for all my projects.
- React
- Tailwind CSS
- React Query
- React Router
- Vite
- Vitest
All data for the current game is stored in a React Query cache and Local Storage. This means that the game state is persisted across sessions.
There isn't much technical complexity here. React Query takes most of that away. What a champ of a library!
The frontend for doodle:ai is hosted on Netlify.
I also have an instance of Vercel's og-image-generator running on vercel for this project.
This allows me to create an og-image using the first image for a puzzle for a given day.
The Backend Server
The backend host is simply a Digital Ocean Spaces instance. Spaces are Digital Ocean's blob store that matches AWS S3 APIs (you can use AWS clients to work with Spaces).
I have a CDN in front of the game files to increase performance and reduce cost.
The backend for generating puzzles is actually a NestJS web application. It might seem strange to run this from a web app instead of a CLI tool but I have a bunch of prebuilt libraries for NestJS that make it very, very fast for me to develop new projects with.
Because stable diffusion requires an NVidia GPU, I run the puzzle generation on my gaming PC with 16GB RAM, an SSD and an Nvidia 2080ti 11GB VRAM.
It takes 2-3 minutes to create a single puzzle. This means around 18 hours for a year of puzzles. Because I'm tweaking the algorithms at the moment, I only generate a few weeks in advance right now. I'll probably generate a year in advance once I'm happy with the algorithms.
Optimised stable diffusion models could probably do a puzzle in 30 seconds and this is something I'm investigating.
The web server acts as the cron manager for the game's Twitter account - @PuzzleAI. This is a bot that posts the first image for a puzzle each day to twitter from NestJS.
For this role the server is running on the same $5 Digital Ocean droplet that I use for all my projects.
The generation APIs are not exposed on this server (no endpoints are public). So it just sits there running cron. It would be easy to move the workers (NestJS application) to a cloud GPU instance in the future to free up my PC, but these GPU-enabled Cloud VMs are quite pricey.
There is a standard conda
environment on my machine which has all the dependencies for image generation using stable diffusion.
Summary
I'm really happy with how Doodle:ai has turned out. It's been a lot of fun to work on and I've learned a lot.
The game stayed on the front page of Hacker News for a full day and I've had a lot of positive feedback from people who have played it.
The game provides a nice platform, and a reason for me to keep learning and improving my knowledge of tools like Stable Diffusion.
Play the game at doodleai.darraghoriordan.com and let me know if you like it!